2009-12-19
2009-12-13
Galton's quincunx in R
Andrej Blejec has a very nice R demo of Galton's quincunx (link1, link2). It is a bit tedious to copy the code from PDF to R console, but it is worth the effort as the demo simulation clearly shows the nature of quincunx. After a bit of search I also found a similar function quincunx in the animation R package by Yihui Xi.
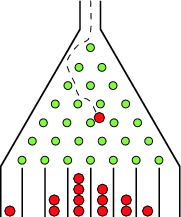
2009-11-20
Components of genetic improvement
I caught a quote (by Blasco) at the ACTEON maling list: "The genetic improvement applied is 15% science and 85% sociology." - translated by Google
2009-11-16
Phenotype MicroArrays - building the hierarchy in biology
I got an e-mail in my box about the presentation of Phenotype MicroArrays (PM) at our department. I will not be able to attend it, but this site describes the idea behind it. This "tool" is a nice addition to a set of DNA/RNA and protein arrays. Adding PM means that we can move one layer above the gene-protein layer. However, as my interest often lies in ultimate phenotype of an individual that we observe, we are still some layers far from deciphering the role of genes on the ultimate phenotype.

2009-11-15
Slick correlation "plots"
Slick correlation "plots" in Wikipedia entry for correlation. I just wonder if correlation should really be zero for the (3,2)-th case. I woukd say it should be slightly more than zero.

2009-11-12
Sweave-Lyx from terminal on Mac
Mark Heckmann writes:
# Find the lyx binary
$ which lyx
/usr/bin/lyx
# Start lyx from console
$ lyx&
In your paper "Using Sweave with Lyx" (great work bty) you pointed out that one can see the sweave error code when processing when starting lyx from the terminal. I just changed from Windows to Mac so that's new for me. Could you send me a few lines how to do that, that is how to operate lyx from terminal and sweave the content from the terminal.I am not familiar with Mac, though would be happy to own one;) Since Mac OS is build on top of some linux/unix like system you need to start the terminal (console) with shell and find the lyx binary. Perhaps something like this on my linux box (text following $ are shell commands)
# Find the lyx binary
$ which lyx
/usr/bin/lyx
# Start lyx from console
$ lyx&
2009-10-13
Free copy of book by Hastie, Tibshirani, and Friedman
Hastie and Tibshirani mentioned in their course in Krems am Donau (Austria) that the last edition of their book "The Elements of Statistical Learning: Data Mining, Inference, and Prediction" will be freely available. It is true! You can fetch a free copy from Hastie's webpage (8.2 MB).
RExcel
I am not a fan of MS Windows software. However, the reality is that majority of people use it and it is often easier for me to live with this than to convert the sorounding souls. Additionally I am find ing out lately that majority of my favourite open-source programs work on all major platforms: MS Windows, Mac, and UNIX/Linux. Then there is no problem for me to work on any of these systems. MS Excel tool is similarly very widely used by mases, but not really powerfull when it comes to data analysis. However, we must admit that it is jolly usefull for handling medium sized data and that majority of people perform analyses with Excel. For those that can not live without Excel and have some working knowledge of R I suggest to take a look at the RExcel and its demo video (28 min!). It seems to be very nicely integrated - Excel keeps tracks of all the dependencies, while R takes care of the computations. Of course you can do the same stuff in R, by reruning the script for each change, but the interactivity offered by the Excel has its own merit. Regarding the quality of graphics it is obvious that Excel plots can not match with R. But if you need some interactivity (imagine you would like to study the effect of a particular parameter on distribution density), then Excel plots are good enough.
2009-10-07
2009-10-02
smoothScatter in base R
smoothScatter function is now available also in base R - in the recommended package graphics that is shipped with R. Originally this function was in the genepplotter package from Bioconductor. I really like it since it can nicely plot large datasets. Bellow is an example figure (also available at R Graph Gallery). Simply start R and type ?smoothScatter to get familiar with this function.
New version of Pedigree Viewer
Brian and Sandy Kinghorn have made quite some changes to the popular Pedigree Viewer program. In particular they added the mate-selection module. I definitely have to try it out!
2009-09-22
Sustainable selection on body weight in minks
In several species breeders want to increase the body weight to obtain more efficient animals. Often animals are fed ad libitum to give the opportunity to animals to show their growth (as well as obesity) to a maximal extent. However, Danish scientist reported that this might not be sustainable - at least for minks. They conducted the experiment with restricted and ad libitum feeding regime and found out that minks from the restricted group were more fertile and had better utilization of feed. I wonder how does this relate to pigs, rabbits, etc.
2009-09-21
Gelmans talk at Applied Statistics conference in Ribno (Slovenia)
I had an oppurtunity to attend the talk by the Andrew Gelman at the Applied Statistics conference in Ribno (Slovenia). It was nice to meet him "in flesh" after reading his books and blog for quite some time now. He is more "funny" and relaxed than I anticipated, but his talk was very brilliant, He mainly talked about weakly informative prior - his new secret weapon ;) Some older slides about this topic are available here.
2009-09-20
ASD talk - Growth performance of station tested rams in Slovenia
I attended the 17th ASD congress in Abano (Italy). I had a presentation about our model development for genetic evaluation of performance tested rams on test stations in Slovenia.
Growth performance of station tested rams in Slovenia
SGD Talk - Fitting pedigree based mixed models in BUGS software
I have a talk today at the 5th SGD congress about fitting animal model (mixed/hierarchical/multilevel model with pedigree information) in BUGS. The abstract was posted before, while the talk slides are available bellow.
Fitting pedigree based mixed models in BUGS software
2009-09-10
Changing font size of R output in Lyx Sweave
Is there a simple way of reducing the size of the font of the R output in Lyx/Sweave? It should be simple, but I can't get anything to work. Placing\huge or \tiny in front of code chunk doesn't change the output.
My reply:
The trick is in what Sweave actually does. Sweave replaces the R code with the code and results, but as Sinput and Soutput environments, i.e.,<<>Becomes>=
1 + 1
@\begin{Schunk}Therefore, I think you need to modify the Sinput and/or Soutput tex environments
\begin{Sinput}
> 1 + 1
\end{Sinput}
\begin{Soutput}
[1] 2
\end{Soutput}
\end{Schunk}
defined in the Sweave.sty file. However, I have never done anything like that. I think there are some R packages on CRAN that did some of this things - I remember seeing green output etc.
2009-09-04
Povzetek Interbull srečanja v Barceloni
Od 22. do 23. avgusta sem se udeležil konference Interbull v Barceloni. Spodaj je povzetek pomembnejših prispevkov. Celotni prispevki pa so na voljo na spletni strani.
Tema: Nacionalni obračuni
V tem sklopu so bile predstavljene novosti, posebnosti, … iz nacionalnih obračunov plemenskih vrednosti.
• Genetic evaluation for fertility traits in Austria and Germany (Fuerst in Gredler)
Avstrijci so za populacijo lisastega in rjavega goveda predstavili nov pristop k analizi plodnosti krav, kjer so vključili pet lastnosti: NR56 ločeno za prvesnice in ostale krave, število dni od telitve do prve osemenitve in število dni od prve do zadnje osemenitve ločeno za prvesnice in ostale krave (FLI). S tem so zamenjali stari pristop z eno samo lastnostjo NR90. Plemenske vrednosti posameznih lastnosti (le NR56 in PZ ločeno za obe skupini) združijo najprej v indeks za plodnost (1/8*NRp + 3/8*NRk + 1/8*FLIp + 3/8*FLIk) in nato še v skupni selekcijski indeks, kjer je teža za »plodnost« ekonomsko ovrednotena in znaša 6,8% pri lisasti in 8,6% pri rjavi pasmi. Predstavili so tudi genetske trende za plodnost, ki ne kažejo negativnih premikov v zadnjih letih, kar je zaželeno.
• Predicting mastitis resistance breeding values from somatic cell count indicator traits (Eding in sod.)
Nizozemci so napravili pilotno študijo, kjer so iskali povezave med spremembami v številu somatskih celic (definirano na različne načine) in sub-kliničnim ter kliničnim mastitisom. Rezultate te študije sedaj uporabljajo pri nacionalnih obračunih, kjer imajo na voljo samo podatke o številu somatskih celic ne pa tudi o incidenci sub-kliničnih ter kliničnih mastitisov. Diskusija je tekla na temo, da ni mogoče in kako bi bilo možno zbrati podatke o kliničnih mastitisih.
• Simultaneous and recursive random regression models for milk yield and somatic cell score in Canadian Holsteins (Jamrozik in sod.)
Pri kravah z večjo količino mleka so mastitisi pogostejši. Standardni modeli za genetsko vrednostenje upoštevajo to povezavo, a pri tem ni jasno kaj je vzrok in kaj posledica. Skupina raziskovalcev iz Kanade je uporabila naprednejši model, kjer je možno testirati kaj je vzrok in kaj posledica. Rezultati njihove analize so pokazali, da povečana mlečnost rahlo povečuje število somatskih celic, medtem ko povečano število somatskih celic močno znižuje prirejo mleka. S tem so pokazali, da selekcija na večjo količino mleka ob spremljanju števila somatskih celic ne povečuje števila somatskih celic. Kljub temu, da naprednejši model omogoča testiranje vzročnih povezav, se rangiranje živali v primerjavi s standardnim modelom ni razlikovalo.
• Estimation of parameters for heterogeneous variance adjustment on test-day data (Márkus in sod.)
Teoretična in metodološka študija ocene heterogenih varianc (med leti, čredami, …) na simuliranih in realnih podatkih iz Skandinavskih držav.
• Estimation of variance components for Nordic red cattle test-day model: Bayesian Gibbs sampler vs. Monte Carlo EM REML (Lidauer in sod.)
Teoretična in metodološka študija različnih metod za ocenjevanje varianc v modelu, ki ga skupaj uporabljajo Skandinavske države.
• Impact of using reduced rank random regression test-day model on genetic evaluation (Leclerc in sod.)
Države z velikimi populacijami goveda se soočajo z velikimi seti podatkov in s tem posledično velikimi računskimi potrebami za genetsko vrednotenje. Francoska skupina je predstavila eno od možnosti za poenostavitev (redukcijo) statističnega modela, ki je računsko bistveno manj zahtevna a še vedno dovolj natančna.
• Genetic analysis of calf and heifer losses in Danish Holstein (Fuerst-Waltl in Sorensen)
Avstrijska in Danska raziskava je pokazala, da izgube telet in telic do starosti enega leta znašajo okoli 10% in da za to obstaja pričakovano majhen dednostni delež. Izgube po rojstvu so skupno večje kot pa izgube ob rojstvu. Ker je ekonomski pomen teh izgub znaten, se nakazuje možnost za izboljšanje tudi preko selekcije.
• Best use of conventional EBV of bull dams and combination with direct genomic values (Rensing in sod.)
Nemška skupina je predstavila enostavno študijo, s katero so pokazali, da je plemenska vrednost (PV) mladih bikov (telet), ki še nimajo rezultatov hčera, praviloma znatno precenjena. Sklepajo, da je to zaradi precenjenih PV bikovskih mater, saj imajo PV bikovskih očetov praviloma visoke točnosti. Precenjene PV bikovskih mater so najvrjetneje zaradi posebne nege rejcev. Predstavniki iz Nove Zelandije in Nizozemske so potrdili, da so pri njih opazili podobno situacijo. Precenitev PV mladih bikov je problematična, saj so le ti odbrani na podlagi te vrednosti. To posledično vodi do manjšega napredka. Za to Nemci za te živali upoštevajo PV le po očetovi strani. Problem precenjenih PV za mlade bike je pomemben tudi pri genomski selekciji, ker sta pričakovana plemenska vrednost (povprečje staršev) in SNP genotip edina vira informacij za PV mladih bikov.
• Multi-breed genetic evaluation for docility in Irish Suckler Beef Cattle (Evans in sod.)
Rezultati iz Irske kažejo, da je dednostni delež za agresivnost pri mesnih pasmah razmeroma velik. Podatke o agresivnosti zbirajo ocenjevalci kakor tudi rejci. Zbiranje podatkov je obvezno.
• Prediction of beef carcass cut yields using Video Image Analysis (Pabiou in sod.)
Na Irskem ocenjujejo kakovost večine klavnih trupov elektronsko od leta 2005. Pri tem je za vsak trup shranjena tudi slika v elektronskem formatu in shranjena v podatkovno bazo. V predstavljeni študiji so na manjšem setu trupov in naredili disekcijo in ocenili enačbe za napoved mase različnih prodajnih kosov mesa iz zbranih slik. Razvite enačbe bodo nato uporabili za oceno mase prodajnih kosov na liniji klanja in s tem pridobili fenotipske podatke za genetsko vrednotenje.
Tema: Mednarodni obračuni
• Joint Nordic Genetic Evaluation of Growth and Carcass traits in Dairy Breeds (Johansson in sod.)
Skandinavske države so testno izvedle genetsko vrednotenje pitovnih in klavnih lastnosti pri mlečnih pasmah goveda, saj pitanje telet prinaša nekaj dohodka. V analizo so vključili neto dnevni prirast kot dve lastnosti (prirast do starosti 550 dni in prirast nad starostjo 550 dni) in oceno klavnega trupa – omišičenost in zamaščenost. Ocenjeni dednostni deleži so bili v skladu z literaturo. Presenetljive so bile zelo visoke genetske korelacije med prirastom v dveh obdobjih.
• Beef without borders: genetic parameters for Charolaise and Limousine Interbeef genetic evaluation of weaning weights (Venot in sod.)
• Interbeef genetic evaluation of weaning weights for Charolaise and Limousine breeds (Venot in sod.)
• Genetic structure of the European Limousine metapopulation using pedigree analyses (Bouquet in sod.)
V okviru projekta Interbeef je bil razvit sistem genetskega vrednotenja za pasmi šarole in limuzin v Franciji, Danski, Irski, Švedski in Veliki Britaniji. Predstavljeni so bili rezultati, ki so pokazali, da so prednosti mednarodnega vrednotenja za mesne pasme manjši, ker so genetske povezave med državami manjše. Za omenjeni pasmi so genetske povezave med pari držav praviloma nične razen za pare s Francijo. Tako imajo od skupnega vrednotenja predvsem države z manjšimi populacijami (vse razen Francija). Prednost za Francoze je na drugi strani večja populacija in s tem povečana možnost za izogibanje parjenja v sorodstvu.
• Inbreeding rates in breeding programs with different strategies for using genomic selection (Sorensen in Sorensen)
Pri nekaterih mlečnih pasmah goveda je zaradi intenzivne selekcije znaten delež parjenj v sorodstvu. Genomska selekcija sicer prinaša nekaj prednosti, saj je pri tem zmanjšan pomen rodovnikov, a po drugi strani omogoča krajši generacijski interval in s tem dolgoročno večji genetski napredek in večjo stopnjo inbridinga. Danska raziskovalca sta izvedla simulacijo in skušala ugotoviti, kakšna sta genetski napredek in inbriding v primeru uporabe genomske selekcije. Na podlagi rezultatov sta predlagala, da bi se vsi odbrani biki enakomerno uporabljali ne glede na njihovo PV. S tem bi zmanjšali genetski napredek le za malo, hkrati pa bistveno zmanjšali stopnjo inbridinga. Njihov predlog se sicer zdi nenavaden, a gre za dejstvo, da nekoliko milejša selekcija ohranja genetsko variabilnost, ki je potrebna za dolgoročen napredek.
• Dairy cattle fit for diverging purposes (Montgomerie)
Predstavnik iz Nove Zelandije je pripravil provokativno predstavitev, v kateri je izpostavil dobre lastnosti krav pasme Jersey (J) – majhna telesna masa in relativno dobra mlečnost z izjemnim odstotkom maščobe in beljakovin. Na Novi Zelandiji je to druga najpomembnejša mlečna pasma, takoj za črno-belo (HF), in služi za vzrejo križank (JxHF). Kljub temu pasma Jersey v svetovnem merilu izgublja na pomenu. Ker je velik del mleka namenjen predelavi in ne direktni konzumaciji, je izpostavil, da bi morale rejske organizacije in mlekarne bolj sodelovati pri postavitvi cen in rejskih ciljev, saj ni smisel v pridelavi »vode« v mleku.
• Changes in selection of Italian Holsteins (Canavesi in sod.)
Predstavljene so bile novosti/spremembe pri genetske vrednotenju črno-bele pasme v Italiji. Bistvene spremembe so vpeljava več-lastnostnega modela za telesne ocene. Zaradi negativnih genetskih trendov za plodnost so povečali ekonomsko težo za to lastnost in se zaradi tega odpovedali delu genetskega napredka pri lastnosti mlečnosti.
Tema: Uporaba genomskih informacij pri genetskem vrednotenju
• Genomic conversion equations (Loberg in sod.)
Vpeljava genomske selekcije je povzročila nastanek nove skupine živali (mladi biki), za katere je potrebna mednarodna primerjava. V tej študiji so pri Interbull-u ocenili, kako so se spremenile korelacije med državami po uvedbi genomskih informacij. Sklenili so, da spremembe niso znatne in da vpeljava genomske selekcije ne spreminja korelacji med državami.
• Development of Genomic GMACE (Sullivan in VanRaden)
Predstavljena je bila metodologija za mednarodno primerjavo bikov, ki imajo poleg rodovnika in plemenskih vrednosti iz nacionalnih obračunov na voljo še t.i. genomsko plemenskih vrednost. Bik, ki je genotipiziran, s tem pridobi enako količino informacij kot bi jih prispevalo dvajset potomk.
• Relation between accuracies of genomic predictions and ancestral links to the training data (Lund in sod.)
Danski raziskovalci so pokazali, da so točnosti genomskih plemenskih vrednosti odvisne od »oddaljenosti« seta podatkov, ki je bil uporabljen za oceno učinka posameznih SNP označevalcev. Posledično so točnosti praviloma precenjene.
• Evidence of a bias in national evaluation due to genomic preselection (Percy in Ducrocq)
Standardne metode za genetsko vrednotenje v veliki meri odstranijo vpliv selekcioniranih podatkov, če so na voljo (fenotipski) podatki, ki so bili osnova za selekcijo. V primeru genomske selekcije so mladi biki odbrani na podlagi informacij staršev in lastnega genotipa. Tako v nacionalni obračun niso vključeni fenotipski podatki, ki bo omogočilo korekcijo za selekcionirane podatke. Posledično so ocene PV v nacionalnih obračunih podcenjene. Podcenjevanje se povečuje iz leta v leto. Posledično so tudi genetski trendi podcenjeni. Trenutno še ni jasno, katera rešitev je najbolj primerna.
• A simple way to combine genomic and classical information in national BLUP evaluations (Ducrocq in Liu)
• A simple method for correcting the bias caused by genomic pre-selection in conventional genetic evaluation (Liu in sod.)
Obe študiji sta predstavili način, kako bi lahko odstranili vpliv selekcioniranih podatkov. Noben pristop ni optimalen, a odstrani podcenitev v veliki meri.
• National genomic evaluations without genotypes (Harris in sod.)
Na Novi Zelandiji seme distribuirata dve podjetji. Obe podjetji sta investirali v genotipizacijo bikov in nista pripravljeni objaviti genotipov. Zato je računski center pripravil aplikacijo za izračun genomske matrike sorodstva. Obe podjetji pošljeta v računski center matrike namesto genotipov in tako obidejo problem »skrivanja« podatkov.
• Incorporation of correlation between SNPs into the genomic evaluation model (Szyda in sod.)
Nekateri SNP označevalci se nahajajo blizu na kromosomu. Zaradi tega lahko pričakujemo, da bi vključitev te informacije (korelacije) povečala točnost genomskih plemenskih vrednosti. Poljska skupina je preizkusila to idejo, a se je izkazalo, da je takšen model veliko slabši kot standardni pristop, kjer se korelacije med SNP označevalci ne označujejo.
• Estimating reliabilities of genomic breeding values (Calus in sod.)
Raziskovalci iz Nizozemske so predstavili študijo, kjer so pokazali, da so točnosti genomskih plemenskih vrednosti s sedanjimi metodami praviloma precenjene.
• Genomic evaluation testing environment (Jorjani)
Jorjani je razvil program za simulacijo genomskih podatkov, kar bo služilo za primerjavo in razvoj metod. Njegov program (izvorna koda in delujoč program) bo prosto dostopen pri http://genomicselection.net
• Genomic Selection in Simmental/Fleckvieh - First Results (Gredler in sod.)
Avstrijci so predstavili rezultate analiz genomskih podatkov pri bikih lisaste pasme. Uporabili so enake metode kot drugi raziskovalci pri črno-beli pasmi. Njihovi rezultati so nekoliko manj obetavni kot pri črno-beli pasmi. Razlog je lahko v manjši velikosti populacije in manjšem neravnotežju zaradi povezave, kar je lahko posledica manj intenzivne selekcije kot pri črno-beli pasmi. Omenili so, da sodelujejo z Nemci in da bodo v prihodnosti združili podatke in napravili skupno analizo.
• Canadian Implementation of Genomic Evaluations (Doormaal in sod.)
Kanadčani so predstavili, kako so vpeljali genomsko selekcijo. Z ZDA si v celoti delijo podatke, metode in programsko opremo. V Kanadi so rejci intenzivno začeli z genotipizacijo krav – rejci menijo, da če krava ni genotipizirana, ne spada več v skupino elitnih krav. Opravili so tudi oceno SNP označevalcev s setom podatkov samo za bike in nato še za bike in krave. Rezultati so pokazali, da vključitev krav ne izboljša oceno SNP označevalcev. Tako so v tem delu vključeni le biki. Se pa za genotipizirane krave v izračun plemenske vrednosti vključijo poleg rodovnika, lastne prireje in prireje potomk še genomska informacija (SNP označevalci).
• Implementation of genomic evaluation in German Holsteins (Reinhardt in sod.)
Nemci (VIT Verden) so genotipizirali znatno število bikov (nekaj tisoč) in razvili vso potrebno programsko opremo za vpeljavo genomske selekcije. Pri uporabi metod so sledili Američanom.
• Implementation and uptake of genomic evaluations in Ireland (Kearney in sod.)
Irci so zelo ekspeditno vpeljali genomsko selekcijo in so letos že distribuirali seme mladih bikov odbranih na podlagi genomske plemenske vrednosti (GPV). Ker pa imajo GPV nižjo točnost, so rejcem predlagali, da naj raje uporabljajo večje število bikov – vsaj tri ali štiri – napravili so skupine bikov, skupina za mlečnost, skupina za plodnost, … Rejci so upoštevali njihove nasvete v veliki meri.
• Work in progress on Italian Holstein genomic evaluation (van Kaam in sod.)
V Italiji trenutno poteka večje število genomskih projektov pri govedu in drugih gospodarsko pomembnih vrstah domačih živali. Ker je pri črno-beli pasmi vključeno veliko število osemenjevalnih centrov, bodo najbrž biki te pasme genotipizirani zadnji, saj imajo težave z dogovori in lastništvom podatkov. Drugače ima računski center iz Cremone razvite potrebno programsko opremo za implementacijo genomske selekcije.
• Experiences in validating genomic evaluations (Kistemaker in Sullivan)
Kanadska predstavnika ste izpostavila pomen nižje točnosti pri mladih bikih odbranih s pomočjo rodovnika (povprečje plemenskih vrednosti staršev) in genomskih informacij. Ti biki imajo nižje točnosti kot progeno-testirani biki. Ker pa so mlajši, omogočajo večji genetski napredek na leto. Predlog za rejce je tako, da se morajo zavedati tega in da je priporočljivo uporabljati večje število bikov kot do sedaj.
• Utility of unsymmetric mixed model equations for MACE with genomic information (Misztal in sod.)
Sedanji postopek obračunov za genomsko selekcijo je sestavljen iz več faz. Najprej se opravi nacionalni obračun, ki vključuje rodovnike in fenotipske podatke. Nato se del teh rezultatov uporabi v dodatnem obračunu samo za genotipizirane živali, praviloma samo bike. Trenutne metode za ta korak so računsko zelo zahtevne. Nato se oba obračuna združita v t.i. združeno plemensko vrednost. Pri vseh teh korakih je uporabljeno veliko število predpostavk in parametrov, ki jih praviloma ne poznamo. Misztal je predstavil metodologijo, ki omogoča enoten obračun za vse skupine živali – uporabijo se vsi trije viri informacij hkrati: (1) fenotipske vrednosti, (2) rodovniki in (3) SNP označevalci preko genomske matrike sorodstva. Trenutni rezultati kažejo, da je predstavljena metoda uporabna za zelo velike sete podatkov – milijone živali. Metoda še ni popolnoma razvita a obeta novo pot za uporabo genomskih informacij pri selekciji.
Tema: Nacionalni obračuni
V tem sklopu so bile predstavljene novosti, posebnosti, … iz nacionalnih obračunov plemenskih vrednosti.
• Genetic evaluation for fertility traits in Austria and Germany (Fuerst in Gredler)
Avstrijci so za populacijo lisastega in rjavega goveda predstavili nov pristop k analizi plodnosti krav, kjer so vključili pet lastnosti: NR56 ločeno za prvesnice in ostale krave, število dni od telitve do prve osemenitve in število dni od prve do zadnje osemenitve ločeno za prvesnice in ostale krave (FLI). S tem so zamenjali stari pristop z eno samo lastnostjo NR90. Plemenske vrednosti posameznih lastnosti (le NR56 in PZ ločeno za obe skupini) združijo najprej v indeks za plodnost (1/8*NRp + 3/8*NRk + 1/8*FLIp + 3/8*FLIk) in nato še v skupni selekcijski indeks, kjer je teža za »plodnost« ekonomsko ovrednotena in znaša 6,8% pri lisasti in 8,6% pri rjavi pasmi. Predstavili so tudi genetske trende za plodnost, ki ne kažejo negativnih premikov v zadnjih letih, kar je zaželeno.
• Predicting mastitis resistance breeding values from somatic cell count indicator traits (Eding in sod.)
Nizozemci so napravili pilotno študijo, kjer so iskali povezave med spremembami v številu somatskih celic (definirano na različne načine) in sub-kliničnim ter kliničnim mastitisom. Rezultate te študije sedaj uporabljajo pri nacionalnih obračunih, kjer imajo na voljo samo podatke o številu somatskih celic ne pa tudi o incidenci sub-kliničnih ter kliničnih mastitisov. Diskusija je tekla na temo, da ni mogoče in kako bi bilo možno zbrati podatke o kliničnih mastitisih.
• Simultaneous and recursive random regression models for milk yield and somatic cell score in Canadian Holsteins (Jamrozik in sod.)
Pri kravah z večjo količino mleka so mastitisi pogostejši. Standardni modeli za genetsko vrednostenje upoštevajo to povezavo, a pri tem ni jasno kaj je vzrok in kaj posledica. Skupina raziskovalcev iz Kanade je uporabila naprednejši model, kjer je možno testirati kaj je vzrok in kaj posledica. Rezultati njihove analize so pokazali, da povečana mlečnost rahlo povečuje število somatskih celic, medtem ko povečano število somatskih celic močno znižuje prirejo mleka. S tem so pokazali, da selekcija na večjo količino mleka ob spremljanju števila somatskih celic ne povečuje števila somatskih celic. Kljub temu, da naprednejši model omogoča testiranje vzročnih povezav, se rangiranje živali v primerjavi s standardnim modelom ni razlikovalo.
• Estimation of parameters for heterogeneous variance adjustment on test-day data (Márkus in sod.)
Teoretična in metodološka študija ocene heterogenih varianc (med leti, čredami, …) na simuliranih in realnih podatkih iz Skandinavskih držav.
• Estimation of variance components for Nordic red cattle test-day model: Bayesian Gibbs sampler vs. Monte Carlo EM REML (Lidauer in sod.)
Teoretična in metodološka študija različnih metod za ocenjevanje varianc v modelu, ki ga skupaj uporabljajo Skandinavske države.
• Impact of using reduced rank random regression test-day model on genetic evaluation (Leclerc in sod.)
Države z velikimi populacijami goveda se soočajo z velikimi seti podatkov in s tem posledično velikimi računskimi potrebami za genetsko vrednotenje. Francoska skupina je predstavila eno od možnosti za poenostavitev (redukcijo) statističnega modela, ki je računsko bistveno manj zahtevna a še vedno dovolj natančna.
• Genetic analysis of calf and heifer losses in Danish Holstein (Fuerst-Waltl in Sorensen)
Avstrijska in Danska raziskava je pokazala, da izgube telet in telic do starosti enega leta znašajo okoli 10% in da za to obstaja pričakovano majhen dednostni delež. Izgube po rojstvu so skupno večje kot pa izgube ob rojstvu. Ker je ekonomski pomen teh izgub znaten, se nakazuje možnost za izboljšanje tudi preko selekcije.
• Best use of conventional EBV of bull dams and combination with direct genomic values (Rensing in sod.)
Nemška skupina je predstavila enostavno študijo, s katero so pokazali, da je plemenska vrednost (PV) mladih bikov (telet), ki še nimajo rezultatov hčera, praviloma znatno precenjena. Sklepajo, da je to zaradi precenjenih PV bikovskih mater, saj imajo PV bikovskih očetov praviloma visoke točnosti. Precenjene PV bikovskih mater so najvrjetneje zaradi posebne nege rejcev. Predstavniki iz Nove Zelandije in Nizozemske so potrdili, da so pri njih opazili podobno situacijo. Precenitev PV mladih bikov je problematična, saj so le ti odbrani na podlagi te vrednosti. To posledično vodi do manjšega napredka. Za to Nemci za te živali upoštevajo PV le po očetovi strani. Problem precenjenih PV za mlade bike je pomemben tudi pri genomski selekciji, ker sta pričakovana plemenska vrednost (povprečje staršev) in SNP genotip edina vira informacij za PV mladih bikov.
• Multi-breed genetic evaluation for docility in Irish Suckler Beef Cattle (Evans in sod.)
Rezultati iz Irske kažejo, da je dednostni delež za agresivnost pri mesnih pasmah razmeroma velik. Podatke o agresivnosti zbirajo ocenjevalci kakor tudi rejci. Zbiranje podatkov je obvezno.
• Prediction of beef carcass cut yields using Video Image Analysis (Pabiou in sod.)
Na Irskem ocenjujejo kakovost večine klavnih trupov elektronsko od leta 2005. Pri tem je za vsak trup shranjena tudi slika v elektronskem formatu in shranjena v podatkovno bazo. V predstavljeni študiji so na manjšem setu trupov in naredili disekcijo in ocenili enačbe za napoved mase različnih prodajnih kosov mesa iz zbranih slik. Razvite enačbe bodo nato uporabili za oceno mase prodajnih kosov na liniji klanja in s tem pridobili fenotipske podatke za genetsko vrednotenje.
Tema: Mednarodni obračuni
• Joint Nordic Genetic Evaluation of Growth and Carcass traits in Dairy Breeds (Johansson in sod.)
Skandinavske države so testno izvedle genetsko vrednotenje pitovnih in klavnih lastnosti pri mlečnih pasmah goveda, saj pitanje telet prinaša nekaj dohodka. V analizo so vključili neto dnevni prirast kot dve lastnosti (prirast do starosti 550 dni in prirast nad starostjo 550 dni) in oceno klavnega trupa – omišičenost in zamaščenost. Ocenjeni dednostni deleži so bili v skladu z literaturo. Presenetljive so bile zelo visoke genetske korelacije med prirastom v dveh obdobjih.
• Beef without borders: genetic parameters for Charolaise and Limousine Interbeef genetic evaluation of weaning weights (Venot in sod.)
• Interbeef genetic evaluation of weaning weights for Charolaise and Limousine breeds (Venot in sod.)
• Genetic structure of the European Limousine metapopulation using pedigree analyses (Bouquet in sod.)
V okviru projekta Interbeef je bil razvit sistem genetskega vrednotenja za pasmi šarole in limuzin v Franciji, Danski, Irski, Švedski in Veliki Britaniji. Predstavljeni so bili rezultati, ki so pokazali, da so prednosti mednarodnega vrednotenja za mesne pasme manjši, ker so genetske povezave med državami manjše. Za omenjeni pasmi so genetske povezave med pari držav praviloma nične razen za pare s Francijo. Tako imajo od skupnega vrednotenja predvsem države z manjšimi populacijami (vse razen Francija). Prednost za Francoze je na drugi strani večja populacija in s tem povečana možnost za izogibanje parjenja v sorodstvu.
• Inbreeding rates in breeding programs with different strategies for using genomic selection (Sorensen in Sorensen)
Pri nekaterih mlečnih pasmah goveda je zaradi intenzivne selekcije znaten delež parjenj v sorodstvu. Genomska selekcija sicer prinaša nekaj prednosti, saj je pri tem zmanjšan pomen rodovnikov, a po drugi strani omogoča krajši generacijski interval in s tem dolgoročno večji genetski napredek in večjo stopnjo inbridinga. Danska raziskovalca sta izvedla simulacijo in skušala ugotoviti, kakšna sta genetski napredek in inbriding v primeru uporabe genomske selekcije. Na podlagi rezultatov sta predlagala, da bi se vsi odbrani biki enakomerno uporabljali ne glede na njihovo PV. S tem bi zmanjšali genetski napredek le za malo, hkrati pa bistveno zmanjšali stopnjo inbridinga. Njihov predlog se sicer zdi nenavaden, a gre za dejstvo, da nekoliko milejša selekcija ohranja genetsko variabilnost, ki je potrebna za dolgoročen napredek.
• Dairy cattle fit for diverging purposes (Montgomerie)
Predstavnik iz Nove Zelandije je pripravil provokativno predstavitev, v kateri je izpostavil dobre lastnosti krav pasme Jersey (J) – majhna telesna masa in relativno dobra mlečnost z izjemnim odstotkom maščobe in beljakovin. Na Novi Zelandiji je to druga najpomembnejša mlečna pasma, takoj za črno-belo (HF), in služi za vzrejo križank (JxHF). Kljub temu pasma Jersey v svetovnem merilu izgublja na pomenu. Ker je velik del mleka namenjen predelavi in ne direktni konzumaciji, je izpostavil, da bi morale rejske organizacije in mlekarne bolj sodelovati pri postavitvi cen in rejskih ciljev, saj ni smisel v pridelavi »vode« v mleku.
• Changes in selection of Italian Holsteins (Canavesi in sod.)
Predstavljene so bile novosti/spremembe pri genetske vrednotenju črno-bele pasme v Italiji. Bistvene spremembe so vpeljava več-lastnostnega modela za telesne ocene. Zaradi negativnih genetskih trendov za plodnost so povečali ekonomsko težo za to lastnost in se zaradi tega odpovedali delu genetskega napredka pri lastnosti mlečnosti.
Tema: Uporaba genomskih informacij pri genetskem vrednotenju
• Genomic conversion equations (Loberg in sod.)
Vpeljava genomske selekcije je povzročila nastanek nove skupine živali (mladi biki), za katere je potrebna mednarodna primerjava. V tej študiji so pri Interbull-u ocenili, kako so se spremenile korelacije med državami po uvedbi genomskih informacij. Sklenili so, da spremembe niso znatne in da vpeljava genomske selekcije ne spreminja korelacji med državami.
• Development of Genomic GMACE (Sullivan in VanRaden)
Predstavljena je bila metodologija za mednarodno primerjavo bikov, ki imajo poleg rodovnika in plemenskih vrednosti iz nacionalnih obračunov na voljo še t.i. genomsko plemenskih vrednost. Bik, ki je genotipiziran, s tem pridobi enako količino informacij kot bi jih prispevalo dvajset potomk.
• Relation between accuracies of genomic predictions and ancestral links to the training data (Lund in sod.)
Danski raziskovalci so pokazali, da so točnosti genomskih plemenskih vrednosti odvisne od »oddaljenosti« seta podatkov, ki je bil uporabljen za oceno učinka posameznih SNP označevalcev. Posledično so točnosti praviloma precenjene.
• Evidence of a bias in national evaluation due to genomic preselection (Percy in Ducrocq)
Standardne metode za genetsko vrednotenje v veliki meri odstranijo vpliv selekcioniranih podatkov, če so na voljo (fenotipski) podatki, ki so bili osnova za selekcijo. V primeru genomske selekcije so mladi biki odbrani na podlagi informacij staršev in lastnega genotipa. Tako v nacionalni obračun niso vključeni fenotipski podatki, ki bo omogočilo korekcijo za selekcionirane podatke. Posledično so ocene PV v nacionalnih obračunih podcenjene. Podcenjevanje se povečuje iz leta v leto. Posledično so tudi genetski trendi podcenjeni. Trenutno še ni jasno, katera rešitev je najbolj primerna.
• A simple way to combine genomic and classical information in national BLUP evaluations (Ducrocq in Liu)
• A simple method for correcting the bias caused by genomic pre-selection in conventional genetic evaluation (Liu in sod.)
Obe študiji sta predstavili način, kako bi lahko odstranili vpliv selekcioniranih podatkov. Noben pristop ni optimalen, a odstrani podcenitev v veliki meri.
• National genomic evaluations without genotypes (Harris in sod.)
Na Novi Zelandiji seme distribuirata dve podjetji. Obe podjetji sta investirali v genotipizacijo bikov in nista pripravljeni objaviti genotipov. Zato je računski center pripravil aplikacijo za izračun genomske matrike sorodstva. Obe podjetji pošljeta v računski center matrike namesto genotipov in tako obidejo problem »skrivanja« podatkov.
• Incorporation of correlation between SNPs into the genomic evaluation model (Szyda in sod.)
Nekateri SNP označevalci se nahajajo blizu na kromosomu. Zaradi tega lahko pričakujemo, da bi vključitev te informacije (korelacije) povečala točnost genomskih plemenskih vrednosti. Poljska skupina je preizkusila to idejo, a se je izkazalo, da je takšen model veliko slabši kot standardni pristop, kjer se korelacije med SNP označevalci ne označujejo.
• Estimating reliabilities of genomic breeding values (Calus in sod.)
Raziskovalci iz Nizozemske so predstavili študijo, kjer so pokazali, da so točnosti genomskih plemenskih vrednosti s sedanjimi metodami praviloma precenjene.
• Genomic evaluation testing environment (Jorjani)
Jorjani je razvil program za simulacijo genomskih podatkov, kar bo služilo za primerjavo in razvoj metod. Njegov program (izvorna koda in delujoč program) bo prosto dostopen pri http://genomicselection.net
• Genomic Selection in Simmental/Fleckvieh - First Results (Gredler in sod.)
Avstrijci so predstavili rezultate analiz genomskih podatkov pri bikih lisaste pasme. Uporabili so enake metode kot drugi raziskovalci pri črno-beli pasmi. Njihovi rezultati so nekoliko manj obetavni kot pri črno-beli pasmi. Razlog je lahko v manjši velikosti populacije in manjšem neravnotežju zaradi povezave, kar je lahko posledica manj intenzivne selekcije kot pri črno-beli pasmi. Omenili so, da sodelujejo z Nemci in da bodo v prihodnosti združili podatke in napravili skupno analizo.
• Canadian Implementation of Genomic Evaluations (Doormaal in sod.)
Kanadčani so predstavili, kako so vpeljali genomsko selekcijo. Z ZDA si v celoti delijo podatke, metode in programsko opremo. V Kanadi so rejci intenzivno začeli z genotipizacijo krav – rejci menijo, da če krava ni genotipizirana, ne spada več v skupino elitnih krav. Opravili so tudi oceno SNP označevalcev s setom podatkov samo za bike in nato še za bike in krave. Rezultati so pokazali, da vključitev krav ne izboljša oceno SNP označevalcev. Tako so v tem delu vključeni le biki. Se pa za genotipizirane krave v izračun plemenske vrednosti vključijo poleg rodovnika, lastne prireje in prireje potomk še genomska informacija (SNP označevalci).
• Implementation of genomic evaluation in German Holsteins (Reinhardt in sod.)
Nemci (VIT Verden) so genotipizirali znatno število bikov (nekaj tisoč) in razvili vso potrebno programsko opremo za vpeljavo genomske selekcije. Pri uporabi metod so sledili Američanom.
• Implementation and uptake of genomic evaluations in Ireland (Kearney in sod.)
Irci so zelo ekspeditno vpeljali genomsko selekcijo in so letos že distribuirali seme mladih bikov odbranih na podlagi genomske plemenske vrednosti (GPV). Ker pa imajo GPV nižjo točnost, so rejcem predlagali, da naj raje uporabljajo večje število bikov – vsaj tri ali štiri – napravili so skupine bikov, skupina za mlečnost, skupina za plodnost, … Rejci so upoštevali njihove nasvete v veliki meri.
• Work in progress on Italian Holstein genomic evaluation (van Kaam in sod.)
V Italiji trenutno poteka večje število genomskih projektov pri govedu in drugih gospodarsko pomembnih vrstah domačih živali. Ker je pri črno-beli pasmi vključeno veliko število osemenjevalnih centrov, bodo najbrž biki te pasme genotipizirani zadnji, saj imajo težave z dogovori in lastništvom podatkov. Drugače ima računski center iz Cremone razvite potrebno programsko opremo za implementacijo genomske selekcije.
• Experiences in validating genomic evaluations (Kistemaker in Sullivan)
Kanadska predstavnika ste izpostavila pomen nižje točnosti pri mladih bikih odbranih s pomočjo rodovnika (povprečje plemenskih vrednosti staršev) in genomskih informacij. Ti biki imajo nižje točnosti kot progeno-testirani biki. Ker pa so mlajši, omogočajo večji genetski napredek na leto. Predlog za rejce je tako, da se morajo zavedati tega in da je priporočljivo uporabljati večje število bikov kot do sedaj.
• Utility of unsymmetric mixed model equations for MACE with genomic information (Misztal in sod.)
Sedanji postopek obračunov za genomsko selekcijo je sestavljen iz več faz. Najprej se opravi nacionalni obračun, ki vključuje rodovnike in fenotipske podatke. Nato se del teh rezultatov uporabi v dodatnem obračunu samo za genotipizirane živali, praviloma samo bike. Trenutne metode za ta korak so računsko zelo zahtevne. Nato se oba obračuna združita v t.i. združeno plemensko vrednost. Pri vseh teh korakih je uporabljeno veliko število predpostavk in parametrov, ki jih praviloma ne poznamo. Misztal je predstavil metodologijo, ki omogoča enoten obračun za vse skupine živali – uporabijo se vsi trije viri informacij hkrati: (1) fenotipske vrednosti, (2) rodovniki in (3) SNP označevalci preko genomske matrike sorodstva. Trenutni rezultati kažejo, da je predstavljena metoda uporabna za zelo velike sete podatkov – milijone živali. Metoda še ni popolnoma razvita a obeta novo pot za uporabo genomskih informacij pri selekciji.
2009-09-03
Fitting lactation curves/functions in R
It is quite some time ago since I wrote a set of lactation curves/functions in R. I put those functions in the animSci package. However, the package is in a mess for quite some time now - I was adding some new functions, but did not have time to finish the job properly. This is also the reason that package was not published. I got several inquries about the lactation functions. Therefore, I compiled the package and checked that lactation curves work as they should. The package is now published here with a warning.
The key fact to fit a particular lactation curve in R is to create a function that will take a numeric variable (days in milk) and create a design matrix that can be fed to model fitting function such as lm() or similar, e.g.,
someFunc <- function(x, ...) ... lm(y ~ someFunc(x))
It must be noted that this approach is not the most efficient for large datasets, since design matrix can be large, but this is a well known problem with using lm() in R. I implemented four lactation functions: Wood, Wilmink, Ali-Schaeffer, and Guo-Swalve, but others can be added. Bellow is the R code to run the lactation curves example on a data set of four milk traits in goats and the resulting figure. It would be interesting to compare this functions with Legendre polynomials (see here) and splines.
## Add my repository
tmp <- c("http://gregor.gorjanc.googlepages.com",
contrib.url(repos=getOption("repos"),
type=getOption("pkgType")))
## Install the package
install.packages("animSci", contriburl=tmp, dep=TRUE)
## Load the package
library(package="animSci")
## Run the lactation curve examples
example(topic="lactCurves")
P.S. I got a nice cover up from David Smith.
Some lactation curves for milk traits in goat
2009-08-26
EAAP Talk: Inference of genotype probabilities and derived statistics for PrP locus in sheep
I had a talk (see bellow) today at 60th EAAP meeting in Barcelona at the Sheep and Goats free communications session. Details of the talk are in the paper (a shortened version will appear on the EAAP site in the following days, but you might prefer the long version instead). I got some nice responses which is great. I have to mention that EAAP granted me a scholarship to present our results. This is greatly appreciated. I will post more about the trip to Barcelona in few days.
Inference of genotype probabilities and derived statistics for PrP locus in sheep
2009-08-08
What is so civil about the war, anyway?
I am a fan of Guns & Roses band. Well, this dates back to being a teenager, but I still like their songs. Today I was in mood and listen to a bunch of their songs, and it only occured to me now about the meaning of what Axl says at the end of "Ciwili war" song. He says. "What is so civil about the war, anyway?". Good point!
Open access
PhD comics has a very nice comic on "Nature vs Scince vs Open Access". It is a nice comic that points out some problems with current system of publishig our work.
2009-08-07
Additive vs. dominance two-allele genetic model by DIC
Two-allele model has alleles A1 and A2 and genotypes A1A1, A1A2, and A2A2. This model can be fitted using only additive effect (restricted model) or additve+dominance effect. (full model) The later model has one parameter more than the former. I was using this model lately and got weird DIC results - the full model had less number of parameters. Bellow is a simulation (using R and BUGS), that shows expected DIC results.
## Needed packages and functions
library(package="e1071")
library(package="doBy")
library(package="R2WinBUGS")
rvalue <- function(n, p, value, mean=0, sdE=0)
{
if(!is.matrix(value)) value <- as.matrix(value)
## Frequencies
k <- length(p)
q <- 1 - p
P <- p * p
Q <- q * q
H <- 2 * p * q
## Allocate matrix of arbitrary values by loci
x <- matrix(data=1, nrow=n, ncol=k)
y <- matrix(data=1, nrow=n, ncol=k)
## Simulate arbitrary values
for(i in 1:k) {
x[, i] <- rdiscrete(n=n, probs=c(P[i], H[i], Q[i]))
y[, i] <- value[x[, i], i]
}
x <- x - 1 ## convert 1:3 to 0:2, i.e., number of A1 alleles
## Sum all values
y <- rowSums(y)
## Add mean and normal deviate (error)
y <- y + rnorm(n=n, mean=mean, sd=sdE)
return(as.data.frame(cbind(y, x)))
}
prepairData <- function(x, dominance=FALSE)
{
ret <- list()
ret$n <- nrow(x)
ret$y <- x$y
if(!dominance) {
ret$x <- x$V2 - 1 ## scale to the mean
} else {
ret$x <- x$V2 + 1 ## BUGS does not allow zero indexing!
}
ret
}
### SIMULATE DATA
###-----------------------------------------------------------------------
## --- Purely additive ---
set.seed(seed=19791123)
## Model parameters
n <- 1000
mu <- 100
sigma <- 1
value <- c(-1, 0, 1)
p <- 0.5
## Simulate
podatkiA <- rvalue(n=n, p=p, value=value, mean=mu, sdE=sigma)
par(mfrow=c(2, 1))
hist(podatkiA$y, xlab="Phenotype")
plot(podatkiA$y ~ jitter(podatkiA$V2), xlab="Genotype", ylab="Phenotype")
tmpA1 <- lm(podatkiA$y ~ podatkiA$V2)
abline(coef(tmpA1), lwd="2", col="blue")
tmpA2 <- summaryBy(y ~ V2, data=podatkiA)
points(tmpA2$y.mean ~ tmpA2$V2, pch=19, col="red")
## --- Additive + Dominance ---
set.seed(seed=19791123)
## Model parameters
n <- 1000
mu <- 100
sigma <- 1
value <- c(-1, 1, -1)
p <- 0.5
## Simulate
podatkiD <- rvalue(n=n, p=p, value=value, mean=mu, sdE=sigma)
par(mfrow=c(2, 1))
hist(podatkiD$y, xlab="Phenotype")
plot(podatkiD$y ~ jitter(podatkiD$V2), xlab="Genotype", ylab="Phenotype")
tmpD1 <- lm(podatkiD$y ~ podatkiD$V2)
abline(coef(tmpD1), lwd="2", col="blue")
tmpD2 <- summaryBy(y ~ V2, data=podatkiD)
points(tmpD2$y.mean ~ tmpD2$V2, pch=19, col="red")
### ESTIMATE MODEL PARAMETERS AND DIC
###-----------------------------------------------------------------------
modelA <- function()
{
## --- Additive model (regression on gene content) ---
## Phenotypes
for(i in 1:n) {
y[i] ~ dnorm(mu[i], tau2)
mu[i] <- a + b * x[i]
}
## Location prior
a ~ dnorm(0, 1.0E-6)
b ~ dnorm(0, 1.0E-6)
## Variance prior
lsigma ~ dunif(-10, 10) ## sigma between 4.539993e-05 and 2.202647e+04
tau2 <- pow(sigma, -2)
sigma <- exp(lsigma)
}
modelD <- function()
{
## --- Additive + Dominance model (genotype as a factor) ---
## Phenotypes
for(i in 1:n) {
y[i] ~ dnorm(mu[i], tau2)
mu[i] <- g[x[i]]
}
## Location prior
g[1] ~ dnorm(0, 1.0E-6)
g[2] ~ dnorm(0, 1.0E-6)
g[3] ~ dnorm(0, 1.0E-6)
## Variance prior
lsigma ~ dunif(-10, 10) ## sigma between 4.539993e-05 and 2.202647e+04
tau2 <- pow(sigma, -2)
sigma <- exp(lsigma)
}
tmpAA <- prepairData(x=podatkiA)
tmpAD <- prepairData(x=podatkiA, dominance=TRUE)
tmpDA <- prepairData(x=podatkiD)
tmpDD <- prepairData(x=podatkiD, dominance=TRUE)
initsA <- function() list(a=rnorm(n=1, mean=mu), b=rnorm(n=1), lsigma=0)
initsD <- function() list(g=rnorm(n=3, mean=mu), lsigma=0)
(fitAA <- bugs(model=modelA, data=tmpAA, inits=initsA,
n.chains=3, n.burnin=5000, n.iter=10000, n.thin=10,
bugs.seed=19791123, parameters=c("a", "b", "sigma"),
bugs.directory="C:/Programs/BUGS/WinBUGS14_orig"))
## mean sd 2.5% 25% 50% 75% 97.5% Rhat n.eff
## a 99.9 0.0 99.9 99.9 100 100 100.0 1 1500
## b 1.0 0.0 0.9 1.0 1 1 1.1 1 1500
## sigma 1.0 0.0 1.0 1.0 1 1 1.0 1 400
## deviance 2828.3 2.4 2826.0 2826.0 2828 2829 2834.0 1 1500
## pD = 2.9 and DIC = 2831.2
(fitAD <- bugs(model=modelD, data=tmpAD, inits=initsD,
n.chains=3, n.burnin=5000, n.iter=10000, n.thin=10,
bugs.seed=19791123, parameters=c("g", "sigma"),
bugs.directory="C:/Programs/BUGS/WinBUGS14_orig"))
## mean sd 2.5% 25% 50% 75% 97.5% Rhat n.eff
## g[1] 98.9 0.1 98.8 98.9 98.9 99 99.1 1 660
## g[2] 99.9 0.0 99.8 99.9 99.9 100 100.0 1 1500
## g[3] 101.0 0.1 100.8 100.9 101.0 101 101.1 1 1500
## sigma 1.0 0.0 1.0 1.0 1.0 1 1.0 1 1300
## deviance 2829.2 2.9 2826.0 2827.0 2829.0 2831 2837.0 1 1500
## pD = 4.0 and DIC = 2833.2
## pD seem OK and DIC agrees with simulated data - it is worse
## for AD model for data following additive model
(fitDA <- bugs(model=modelA, data=tmpDA, inits=initsA,
n.chains=3, n.burnin=5000, n.iter=10000, n.thin=10,
bugs.seed=19791123, parameters=c("a", "b", "sigma"),
bugs.directory="C:/Programs/BUGS/WinBUGS14_orig"))
## mean sd 2.5% 25% 50% 75% 97.5% Rhat n.eff
## a 100.0 0.0 99.9 100.0 100.0 100.0 100.1 1 570
## b 0.0 0.1 -0.1 0.0 0.0 0.1 0.2 1 1500
## sigma 1.4 0.0 1.3 1.4 1.4 1.4 1.5 1 1300
## deviance 3512.9 2.6 3510.0 3511.0 3512.0 3514.0 3520.0 1 1500
## pD = 3.0 and DIC = 3515.9
(fitDD <- bugs(model=modelD, data=tmpDD, inits=initsD,
n.chains=3, n.burnin=5000, n.iter=10000, n.thin=10,
bugs.seed=19791123, parameters=c("g", "sigma"),
bugs.directory="C:/Programs/BUGS/WinBUGS14_orig"))
## mean sd 2.5% 25% 50% 75% 97.5% Rhat n.eff
## g[1] 98.9 0.1 98.8 98.9 99.0 99 99.1 1 1500
## g[2] 100.9 0.1 100.8 100.9 100.9 101 101.0 1 1500
## g[3] 99.0 0.1 98.8 98.9 99.0 99 99.1 1 1500
## sigma 1.0 0.0 1.0 1.0 1.0 1 1.0 1 530
## deviance 2829.2 2.9 2826.0 2827.0 2829.0 2831 2837.0 1 1500
## pD = 4.1 and DIC = 2833.3
## pD seems OK and DIC also agrees with the model --> dominance
## model is better for this dataset
2009-07-30
Euston Grove Press offers to interested users a free download of the book "Sewall Wright Taught Me. 2. Genetics". Here is the link. For me this is interesting, but impossible to read.

2009-07-16
LyX-Sweave: mandatory use of control+enter in code chunks
A user of LyX-Sweave is asking:
My embarrassingly simple question is this: For class notes, I often have lots of code to include. I develop code in R, & paste code into a scrap section. But then I have to step through it all and find the spots to insert control-enter to terminate lines. Inevitably I miss a few, then the code breaks (without a clue as to where). So, is there a simple way to move the code into a scrap that bypasses the control-enter problem?
First of all, you can easily see where Sweave has problem by launching LyX from the terminal. Then you will see the output of R CMD Sweave in the terminal and you can easily spot the problematic chunk. This works on MS Windows as well as on Linux! There is some development going on with LyX and it seems that it will be possible in the future to see the Sweave log in LyX directly as it is possible for LaTeX log.
I agree that mandatory the use of cotnrol+enter in scrap (code chunk) environment is suboptimal. I also hate it! But that is the way LyX is created. One way you can avoid this for now is to not use the "scrap" environment at all - you can insert the code chunks (starting with <<>>= and ending with @) in the LaTeX code (use shortcut control+l). I tried this on my laptop and works without problems. In future versions of LyX this resctriction might be lifted.
Page2RSS
For me e-mail and RSS are two really important components of world-wide-web. However, some sites do not publish RSS, which is a pitty given that I do not have time to visit them often. In such cases Page2RSS service can be used to be informed about sites changes. I find this service today and I hope it will work fine.
2009-07-14
S kozami nad plevel
Lep primer, kako lahko s kozami uspešno "saniramo" zaraščeno površino s pleveli. Uspeh na slikah je kar preveč dober. Če bi imeli bistveno večjo površino, uspeh najbrž ne bi bil tako dober - pri tem je ključna obtežba - za "čiščenje" mora biti obtežba ZELO velika.
2009-07-06
9th WCGALP - next year
The website for the 9th WCGALP (World Congress on Genetics Applied to Life Stock Production) is online. There is not much there yet, but this will surely change with time.
Here is a link to previous WCGALP in Brasil in 2006. I can not find the website of the 7th anymore - it was held in Montpellier (France).
2009-07-03
Evaluation of different approaches for the estimation of daily yield from single milk testing scheme in cattle
Janez finnished and submitted the paper "Evaluation of different approaches for the estimation of daily yield from single milk testing scheme in cattle". He did the majority of job! I improved his work a bit with the comments and restructuring/rewritting some parts of the paper.
Evaluation of different approaches for the estimation of daily yield from single milk testing scheme in cattle
2009-06-27
2009-06-19
bugsparallel
bugsparallel is a Metrum Institute project to run BUGS (via R2WinBUGS) in parallel - McMC is an application, where parallel runs can be used very efficientlly. Here is the code for one example using bugsparallel.
Some usefull links:
Some usefull links:
2009-06-16
Samanthina valeta
2009-06-12
European Summer Institute in Statistical Genetics 2009
... will take place at Univeristy of Liege from Aug. 31 to Sep. 9. The Graphical model for genetics module looks particularly interesting to me, but there are also other very fine modules!
Computational aspects in Animal Breeding
Here is a nice presentation by Misztal and Rekaya about computational aspects in animal breeding.
2009-06-11
2009-06-09
2009-06-04
2009-05-27
Embeding fonts in figures produced by R
Some publishers insist that we embed (include) the fonts in each figure. Here is a set of links regarding this issue for figures produced by R:
Generation interval calculation example
Here is a nice sheet with example of generation interval calculation in a flock of sheep.
2009-05-25
Fitting pedigree based mixed models in BUGS software
Bellow is my abstract for talk at joint congress of SBD and SGD at Otočec. I will show how BUGS (Bayesian Using Gibbs Sampling) software can be used to fit the so called animal model. Now I need to prepair the talk and perhaps even write a short communication for some journal. Added 2009-09-20: The talk is available here.
Pedigree based mixed model (commonly called animal model) is an important class of statistical models for inference of quantitative genetic parameters in various fields such as animal and plant breeding, evolutionary biology and human genetics. In last years Bayesian statistics has been introduced to a set of standard statistical procedures of a quantitative geneticists' toolbox, due to the ever increasing complexity of fitted models. While several specific programs can be used to fit animal model using Bayesian approach, none of them provide and easy to use and flexible environment for the development and testing of models. It is common to use favourite programming language to develop the needed programs, but this requires a considerable amount of programming and statistical skills. A viable alternative is to use general purpose statistical packages. BUGS (Bayesian Using Gibbs Sampling) is a popular and fairly flexible program for the Bayesian analysis of complex statistical models using Markov Chain Monte Carlo methods. Recently two reports of fitting animal model in BUGS were given, but both failed to provide a generic procedure that can be used independently of the collected data. Here, a generic description of animal model is presented using the concept of graphical models. This description was translated to BUGS language and fitted to a small example. Comparison with other programs revealed the validity of a new procedure. Tests with other data sets showed that BUGS can be used to efficiently fit animal model for medium sized data sets. Using these results quantitative geneticists can now easily use Bayesian approach to fit animal model in BUGS.
2009-05-02
Genetic architecture of quantitative traits in mice, flies, and humans
Flint and MacKay published a paper: "Genetic architecture of quantitative traits in mice, flies, and humans". I think it is now is clear that revolutionary ideas that were spured by the field of molecular genetics are still far from reality. There surely is a lot of progress, but also at considerable investments.
Those interested in quantitative genetics might also be interested in:
2009-04-30
SAS goes with sparse matrices
SAS has introduced experimental procedure HPMIXED (High Performance MIXED) in version 9.2. This is a welcome addition and now SAS could probably solve the problem I encountered lately with a large mixed model described here. I really like R and its community (which is getting bigger and bigger and more and more connected to other communities!), but I must say that for me SAS has made quite some important moves lately - see here, here, and here.
2009-04-23
Statistics for Molecular Genetics
Nice notes about Statistics for Molecular Genetics by Karin Dorman.
2009-04-21
Genes for cowboys
I rally like the title of this "material": Genes for cowboys. I still need to parse the content.
2009-04-19
Video posnetki povezani s čebelami
Danes sem naletel na zgledno urejeno spletno stran ČEBELARSTVO Grega Lužar - tipa sploh ne poznam, tako da mu ne delam reklame. Na spletni strani je kar nekaj zanimivih video posnetkov opravil/dogodkov, ki jih sam še nikoli nisem videl:
2009-04-08
Inference for R
I got a message from Inference for R team. Their work is interesting, especially if you are bound to MS Excel and Word environment! It would be great if they would also support OpenOffice. They "benefit" from open source R and it would be great if they would also provide a solution for open source "office".
2009-04-03
2009-04-01
Sweave.sh update
A local version of Sweave.sh has been updated:
- fixed a buglet that caused weaving the file twice when --weaver option was used --> this led to a change how caching is now invoked (--cache invokes cacheSweave package, while --cache --weaver or --weaver invokes weawer package)
- quoting *APP variables to ensure that things work in case of "bad" filenames, i.e., spaces in filenames etc.
- small changes in the documentation
I will take this opportunity to show how PDFAPP and PSAPP environmental variables can be used "open" options. This is an example from the help, which shows that these two variables need to be exported in order to have any effect. I am still fiddling whith how to provide any meaningfull defaults. My defaults are 'acroread' for PDF and 'gv' for Postscript, but I am happy to set any other more meaningfull defaults if there will be enough demand!
# Create PDF via the texi2dvi (latex) tool and open
# a produced file
Sweave.sh -otld file.Rnw
# Create PDF via the texi2dvi (latex) tool and open
# a produced file with a "non-standard" viewer
Sweave.sh -otld=acrobat file.Rnw
# ... or
export PDFAPP=acrobat
Sweave.sh -otld file.Rnw
This is an example of launcing Acrobat Reader installed on MS Windows, while Sweave.sh is launched within Cygwin X-terminal:
export PDFAPP=/cygdrive/c/Program\ Files/Adobe/Reader\ 9.0/Reader/AcroRd32.exe
Sweave.sh -otld file.Rnw
2009-03-31
Semiparametric regression via penalized splines literature
Interested in semiparametric regression via penalized splines? Take a look here.
2009-03-24
Kako ravnajo s poginulimi živalmi čez lužo
Glej "Dealing With Deadstock". Pri nas pa v določenih pogledih pretiravamo do skrajne mere. Se povsem strinjam, da mora biti ta segment živinoreje primerno urejen, a za to izvajati "vesoljske" procese ni razumno.
Genomic selection in beef cattle
Stephen P. Miller has wrote two short articles about application of genomic selection in beef cattle:
2009-03-21
Progress bar in R
Nice summary on how to use progress bars in R. I am posting this here in order to have a note for later searches.
2009-03-20
Weather change - shit!
See here what kind of problems are Australian farmers facing due to weather changes.
2009-03-17
Describing genetic variability
One of paramount interests of quantitative geneticists is the knowledge about how much visible (phenotypic) variability is due to genetic variability. To describe this we usually compute narrow sense heritability h2 = Var(a) / Var(y), where y is phenotypic and a is additive genetic value (see book by Falconer and MacKay). A usual result from analyses is that fitness related traits have much lower heritability (say 0.1 or similar) than morphological traits (say 0.5 or even more). This eventually leads to the conclusion that selection will not be effective for fitness related traits, since there is not much additive genetic variability. However, this can only be partialy true. The heritability can have a small value due to small additive genetic variance and/or large phenotypic variance. It is clear that if there is a lot of environmental variability that the selection can not be the prime force to change the population. However, this still does not mean that we should neglect the genetic variability. David Houle has dealt with this issue and published a paper in Genetics (1992). I have read that paper several times and I always forget the exact conclusion. Well, there is no exact conclusion. However, Houle suggests that heritability is not a good measure of evolvability (ability of population to respond to selection) or variability (strength of forces that maintain and deplete genetic variation). He suggests that standardizing the additive genetic variance with a mean is better, which in turn leads to the suggestion that additive genetic coefficient of variation (CVa = Var(a) / Mean(y)) is a more informative measure than heritability. There is also some more recent work by Houle (his pub. webpage, this looks very interesting), that I need to digest - sometime.
An example: we have two populations A and B (this could also be two different traits!), with means 5 and 10, additive genetic variance 5 and 5, heritabilities 0.2 and 0.2. Based on heritabilities we could conclude that selection will have the same effect in both populations, but this is not true in relative meaning. In population A the additive genetic variance is euqal to the mean, whereas it is only half of the mean in population B. The additive genetic coefficient of variations would be 0.45 and 0.22 for population A and B, respectively. This means that we can achive relatively greater response to selection in population A than in population B.
2009-03-14
Video lectures about genetics (biology)
In addition to YouTube videos about genetics there is also a huge number of video lectures at http://videolectures.net/. There is a lot of material on machine learning, statistics, ..., but also biology - see Introduction to Biology which is a course from MIT. Bellow is a link to first lecture on Genetics given by Lander.

Lecture 6: Genetics 1
Eric Lander
Slick spline plot in SAS
Here is an example of a slick spline (using penalized b-splines) plot in SAS. The plot is created with SGPLOT procedure. I hope SAS will add such "functions" also to model based procedures such as GLM, MIXED, GENMOD, ...
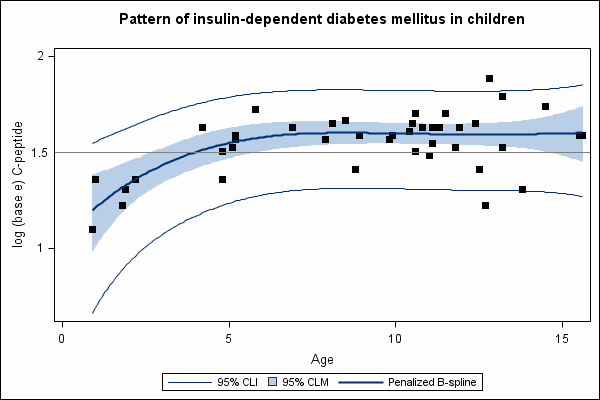
2009-03-13
European Master in Animal Breeding and Genetics
Posredujem novico o štipendijah za študij "European Master in Animal Breeding and Genetics". Kratek pregled predmetnikov (Course contents) na spletni strani http://www.emabg.eu pokaže, da je večina predmetov na temo genetike in selekcije na splošno in ločeno po vrstah živali, ki so pomembne za živinorejo. Je pa na voljo tudi nekaj bolj splošnih predmetov, ki bi lahko bili zelo zanimivi - psi in mačke!
Dear all
Scholarships are available for students from EU countries that want to participate in the European Master in Animal Breeding and Genetics (EMABG) starting in August 2009. Deadlines for applications: May 1st 2009. The deadline for non-EU students that want to participate in course starting this year has passed but they can applied before January 15th 2010 for the next academic year. Information on program and scholarships can be found at www.emabg.eu
The European Master in Animal Breeding is accepted by the European Union as an Erasmus Mundus MSc programme for a five-year period starting in 2007. In 2007, 24 students started the program followed by 21 students in 2008. The universities participating in the EM-ABG are Wageningen University (the Netherlands), University of Natural Resources and Applied Life Sciences (Austria), Christian-Albrechts-Universität (Germany), AgroParisTech (France), Swedish University of Agricultural Sciences (Sweden) and The Norwegian University of Life Sciences (Norway). These groups have a long tradition of collaboration and a strong profile in the area of Animal Breeding and Genetics.
For more information and application, please contact:
EM-ABG secretary, Animal Breeding and Genomics Centre, Wageningen University, PO Box 338, NL 6700 AH Wageningen, The Netherlands, emabg@wur.nl
Kindest regards
Johan van Arendonk
Co-ordinator of EMABG
2009-03-12
YouTube videos about genetics
While browsing for SNP chips for sheep I found out that there are some very nice videos on YouTube about genetics. Here is a list of some, but be free to explore further on!
- Genetics 101 Part 1: What are genes?
- Genetics 101 Part 2: What are SNPs?
- Genetics 101 Part 3: Where do your genes come from?
- Genetics 101 Part 4: What is phenotype?
- Gregor Mendel and Genetics
- Heredity
- Pamela Anderson Illustrates Genetics? - PowerPoint Comedy --> it nicely explains the linkage disequilibrium ;)
Chip for sheep
There is a lot of work in the area of SNP-chips for sheep. See:
- International Sheep Genomics Consortium (YouTube video)
- Australian Sheep Gene Mapping Web Site
- Sheep Genomics
- ...
However, genomic selection with "standard" chips might not be so successful in all sheep populations since there is a lot of variation in a sense that that the linkage disequilibrium is not so strong as in cattle - though I am saying that without having much experience!
2009-03-11
Growth performance of station tested rams in Slovenia
This is our short communication for ASD 2009.
Update 2009-03-13: We had to shorten the manuscript to three pages. This of course lead to the exclusion of some results that will be published elsewhere.
Growth performance of station tested rams in Slovenia
Update 2009-03-13: We had to shorten the manuscript to three pages. This of course lead to the exclusion of some results that will be published elsewhere.
2009-03-10
Substantial update of "Evolution and Selection of Quantitative Traits"
Bruce Walsh send an e-mail to AGDG list about the substantial update of their forthcoming book "Evolution and Selection of Quantitative Traits". After very successful volume 1, we are all eagerly waiting for this volume!
Armidale Animal Breeding Summer Course 2009 Materials
UNE in Armidale organizes each year an Animal Breeding Summer Course. This year they hosted Bruce Walsh (Quantitative Genetic Theory and Analysis: Selection Theory) and Pieter Bijma (Quantitative Genetic Models for social interaction and GxE and inherited variability). What I really like about this course is that all the materials are posted on the website (follow this link for materials for this year). Therefore, all of us that did not had the opportunity to go down under can still study!
2009-03-06
Genomska selekcija
Danes sva imela s Potočnik Klemnom predstavitev o genomski selekciji. Jaz sem na splošno predstavil "klasično" selekcijo in v čem se razlikuje genomska selekcija, medtem ko je Klemen predstavil vtise in informacije z Interbull delavnice. Moj del predstavitve je na voljo tukaj, za pregled stanja pa lahko sledite tej povezavi.
Genomska selekcija
2009-02-22
R graphics: margins are way to large
For me R has a very nice and powerfull capabilities for graphics (for example see this gallery). However, I dislike the default setting for margins and placement of axis numbers and labels. Since I always forget the setting of parameters I prefer I am adding this post. For example:
Now compare this plot to the version I prefer much more:library(package="MASS")
Sigma <- matrix(c(10, 10, 10, 20), nrow=2)
mu <- c(100, 100)
tmp <- mvrnorm(n=200, mu=mu, Sigma=Sigma, empirical=TRUE)
plot(tmp, xlab="X variable (unit)", ylab="Y variable (unit)")
par(bty="l", pty="m", mar=c(3, 3, 1, 1), mgp=c(1.75, 0.75, 0))
plot(tmp, xlab="X variable (unit)", ylab="Y variable (unit)")
Illinois long-term selection experiment for oil and protein in corn
Researchers at the University of Illinois are conducting one of the longest experiments in biology - Illinois long-term selection experiment for oil and protein in corn. The experiment started in 1896 and is still active! In esence they are selecting lines for higher or lower concentration of protein or oil in the kernel. This experiment is very important for a test of the theory of genetics, especially quantitative genetics (link1, link2, link3, link4, link5). I have seen several times the trends from this experiment and I wanted to include them in a talk I am prepairing. A brief search on the web lead me to this site with generation means by line. Bingo! This is all I needed. Bellow is a graph of trends and at the end of the post the R code used to produce the plot.
The theory states that genetic variance and consequently also the genetic gain should diminish after several generations of selection. There are experiments that confirmed that, but in the Illinois corn experiments the limit is not yet reached. Crow (2008) propose the following reasons (verbatim copy!):
- "The environment is continually changing so that what was formerly most fit no longer
is." - "There is an input of genetic variance from mutation, and sometimes from migration."
- "As intermediate-frequency alleles increase in frequency towards one, producing less variance (as p → 1, p(1 − p) → 0), others that were originally near zero become more common and increase the variance. Thus, a roughly constant variance is maintained."
- "There is always selection for fitness and for characters closely related to it."
Now the R code. First I tried to use read.table(file=url(...)), but the data-file had an error - there was a typo on line 68 or 86 - I do not remember anymore. I downloaded the file, fixed the typo and used the following code:
podatki <- read.table(file="corn.txt", na.strings=".", header=TRUE)
cols <- c(rgb(red=204, blue=0, green=0, max=255),
rgb(red=0, blue=153, green=0, max=255),
rgb(red=0, blue=0, green=204, max=255),
rgb(red=204, blue=0, green=153, max=255))
par(bty="l", pty="m", mar=c(5, 4, 1, 1))
matplot(x=podatki$YR, y=podatki[, c("IHP", "ILP", "IHO", "ILO")], type="l", lty=1,
xlab="Year", ylab="Concentration (%)", col=cols[c(1, 1, 2, 2)], lwd=2)
legend("topleft", c("Protein", "Fat"), lty=1,
lwd=2, col=cols[c(1, 2)], bty="n")
2009-02-20
ATLAS
ATLAS (link1, link2) is a Java based tool to manage genotypes. It is handy, but notoriouslly frustrating to install, since there are several different instructions about how to install it - I am not talking about different instructions at different places (say on the net), but in the distribution package. This is very very confusing. I have just spent more than half an hour to install it and I remember I had the same problem last time I tried to install it. The correct instructions are in the ATLAS.html file and this should be done:
- Create ATLAS directory in your user home directory, say "C:\Documents and Settings\GGorjan"
- Add the following files to this folder (Atlas.jar, atlas.ini, chr.atl, ATLASman_v1.4.pdf, ATLAS.html)
- Create directory ATLAS_files such as "C:\Documents and Settings\GGorjan\ATLAS\ATLAS_files" and put all figures and html files in there
2009-02-16
Chromosome - DNA figure
National Human Genome Research Institute has a nice talking glossary of genetic terms. I especially this figure that shows a chromosome and how is it organized, i.e., DNA is wrapped with histones etc. However, there is only one chromosome and this is suboptimal if one wants to show the relationship between the genotype and DNA. Therefore I picked one version from the net (I do not remember where) and changed it a bit. I am publishing it here so that others might benefit as I did. There is plenty of place to annotate it.

2009-02-15
R in SAS
Another "proof" that R definitely is one of mainstream statistical packages is the news that SAS will provide an interface to R via SAS/IML Studio (today known as SAS Stat Studio).
2009-02-11
Fitting Legendre (orthogonal) polynomials in R
Frederick Novomestky packaged a series of orthogonal polynomials in the orthopolynom R package. However, his functions can not be used "directly" in a statistical model, say in lm(). There is no need to use functions from orthopolynom package, since there is a poly() function in stats package that is shipped with R. Nevertheless, I played with class of Legendre polynomials. (Wikipedia, Wolfram) This class of polynomials is very popular in my field since the introduction of so called random regression models (e.g. link1, link2, link3, ..., and some of our work), though the splines (Wikipedia, Wolfram) are making their way through (see this link and follow references). Let's go to orthopolynom package. Say we want to use Legendre polynomial of 4th order for a variable x. First we need to get the coefficients for basis functions:
library(package="orthopolynom")
(leg4coef <- legendre.polynomials(n=4, normalized=TRUE))[[1]]
0.7071068
[[2]]
1.224745*x
[[3]]
-0.7905694 + 2.371708*x^2
[[4]]
-2.806243*x + 4.677072*x^3
[[5]]
0.7954951 - 7.954951*x^2 + 9.280777*x^4
To use this polynomial in a model, we need to create a design matrix with sensible column names and without the intercept:
x <- 1:100
leg4 <- as.matrix(as.data.frame(polynomial.values(polynomials=leg4coef,
x=scaleX(x, u=-1, v=1))))
colnames(leg4) <- c("leg0", "leg1", "leg2", "leg3", "leg4")
leg4 <- leg4[, 2:ncol(leg4)]
Now we can use this in a model, e.g., lm(y ~ leg4). I made this whole process easier - with the functions bellow, we can simply use lm(y ~ Legendre(x=scaleX(x, u=-1, v=1), n=4)). I contacted Fred and I hope he will add some version of these functions to his package.
Update 2009-03-16: If we want that the intercept has a value of 1, we need to rescale the polynomial coefficients by multiplying with sqrt(2).
Update 2009-03-16: If we want that the intercept has a value of 1, we need to rescale the polynomial coefficients by multiplying with sqrt(2).
Legendre <- function(x, n, normalized=TRUE, intercept=FALSE, rescale=TRUE)
{
## Create a design matrix for Legendre polynomials
## x - numeric
## n - see orthopolynom
## normalized - logical, see orthopolynom
## intercept - logical, add intercept
tmp <- legendre.polynomials(n=n, normalized=normalized)
if(!intercept) tmp <- tmp[2:length(tmp)]
polynomial.values(polynomials=tmp, x=x, matrix=TRUE)
}
polynomial.values <- function(polynomials, x, matrix=FALSE)
{
## Changed copy of polynomial.vales from orthopolynom in order
## to add matrix argument
require(polynom)
n <- length(polynomials)
if(!matrix) {
values <- vector(mode="list", length=n)
} else {
values <- matrix(ncol=n, nrow=length(x))
}
j <- 1
while(j <= n) {
if(!matrix) {
values[[j]] <- predict(polynomials[[j]], x)
} else {
values[, j] <- predict(polynomials[[j]], x)
}
j <- j + 1
}
values
}
2009-02-10
Course: Selección genómica
Hayes and Gianola will have a course "Selección genómica" from 25-29 May in Valencia. See here for details.
2009-02-06
Course: Study of resistance mechanisms in animal infectious diseases course
On behalf of Anne-Sophie Lequarré:
A new session of the well quoted course "Study of resistance mechanisms in animal infectious diseases" will be held one last time at the University of Liège, Belgium from the 16 to the 20 of March 2009 .
This one-week course gives a general introduction to the methods for the identification and exploitation of genetic factors in infectious diseases. It explores the interactions between the genetic diversity of the pathogen and the host in their particular environments. The aim is to integrate the insights of the various disciplines to get a better picture of the genetic basis of resistance to major infectious diseases in livestock.
The course can interest breeders in order to optimize the production in presence of harmful pathogens, biologists to better understand the causes and consequences of co-evolution of the host and its pathogens and of course the epidemiologists.
The course will be taught at a level commensurate with a Master of Science's degree but can also serve as an elective course for researchers wishing to better understand analyses found in the scientific literature. Speakers involved are not only renown scientists but also very good teachers.
The course is supported by EADGENE, a European Network of Excellence on Animal Disease Genomics. Registration prices are quite affordable (250 Euros requested for students or academic personnel). More information can be found at (registration dead-line 15th of February):
http://www.eadgene.info/TrainingCareers/TrainingCourses/EADGENEsupportedc
ourses/StudyofResistanceMechanisms/tabid/242/Default.aspx (please copy whole link into browser or go to http://www.eadgene.info, and follow links within the Training section).
A new session of the well quoted course "Study of resistance mechanisms in animal infectious diseases" will be held one last time at the University of Liège, Belgium from the 16 to the 20 of March 2009 .
This one-week course gives a general introduction to the methods for the identification and exploitation of genetic factors in infectious diseases. It explores the interactions between the genetic diversity of the pathogen and the host in their particular environments. The aim is to integrate the insights of the various disciplines to get a better picture of the genetic basis of resistance to major infectious diseases in livestock.
The course can interest breeders in order to optimize the production in presence of harmful pathogens, biologists to better understand the causes and consequences of co-evolution of the host and its pathogens and of course the epidemiologists.
The course will be taught at a level commensurate with a Master of Science's degree but can also serve as an elective course for researchers wishing to better understand analyses found in the scientific literature. Speakers involved are not only renown scientists but also very good teachers.
The course is supported by EADGENE, a European Network of Excellence on Animal Disease Genomics. Registration prices are quite affordable (250 Euros requested for students or academic personnel). More information can be found at (registration dead-line 15th of February):
http://www.eadgene.info/TrainingCareers/TrainingCourses/EADGENEsupportedc
ourses/StudyofResistanceMechanisms/tabid/242/Default.aspx (please copy whole link into browser or go to http://www.eadgene.info, and follow links within the Training section).
Subscribe to:
Posts (Atom)